
Different module compositions, equals different project patterns
Figure a) Project pattern for an ‘optimal’ run from module 1 till 7
This pattern presents a project where the team started with module ‘Set-Up’ and ran through all the modules till the ‘Post Processing’ module as stated in the overview. Diverse teams which include all the recommended roles and who have a lot of experience with ML projects and a very clear handling of the module outcomes might be able to proceed like this.
This pattern presents a project where the team started with module ‘Set-Up’ and ran through all the modules till the ‘Post Processing’ module as stated in the overview. Diverse teams which include all the recommended roles and who have a lot of experience with ML projects and a very clear handling of the module outcomes might be able to proceed like this.
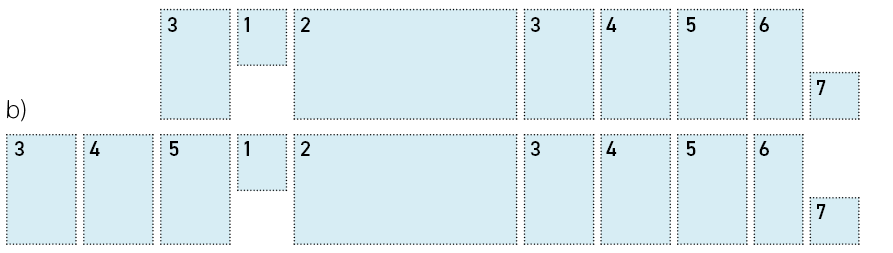
Figures b) Project pattern for initial exploratory data or PoC/Prototyping* phase before project Sst-up
This scenario is very common for ML infused projects. In order to judge whether or not a model’s performance is suitable for the problem at hand, it is usually necessary to train and test the model on a concrete, but small data sample set which has been cleaned and prepared in advance. This takes some time, resources and effort, but on the other hand, is often a necessary validation item before a porper project set-up is made.
This scenario is very common for ML infused projects. In order to judge whether or not a model’s performance is suitable for the problem at hand, it is usually necessary to train and test the model on a concrete, but small data sample set which has been cleaned and prepared in advance. This takes some time, resources and effort, but on the other hand, is often a necessary validation item before a porper project set-up is made.
*As stated before, low fidelity, ‘quick and dirty’ prototyping possibilities for designers, as well as for data scientists and ML engineers are rarely available, as AI/ML algorithms depend on data and user interaction, neither are static, they are dynamic. More concrete a) for this type of generated content, the AI/ML system needs to incorporate pretrained results. Training the model with user testing input would lead to latency in response making the system behavior unacceptable and useless for the user, b) in order to evaluate a system’s performance, the model needs to be trained on actual data that represents a broad range of data points. Training on dummy data or a subset of data could result in misleading model performance and results, c) the architecture that is needed to run a model that generates dynamic content is very difficult to prototype as a low-fidelity solution.

Figure c) Project pattern where ‘Post Processing’ module creates completely new input and modeling needs
Another possible module combination can be derived from the outcome of the ‘Post Processing’ module. If the collected feedback from users creates completely new data points, or if a new feature is requested and retraining a model is not enough, it is possible to set-up a mini project that runs through modules 3 till 5 and is added to the already deployed system. Design expertise involvement might be rather low in that project pattern.
Another possible module combination can be derived from the outcome of the ‘Post Processing’ module. If the collected feedback from users creates completely new data points, or if a new feature is requested and retraining a model is not enough, it is possible to set-up a mini project that runs through modules 3 till 5 and is added to the already deployed system. Design expertise involvement might be rather low in that project pattern.

Figure d) Project pattern where ‘Post Processing’ module results in completely new project
These new data points or feature requests might also result in setting-up a completely new project.
These new data points or feature requests might also result in setting-up a completely new project.